School dates, venue and topics
The school will take place on 24-25 June at Lund University, Sweden. The school is planned to be in-person only, no live streaming will be provided. There will be two courses focusing on different sides of the continuous optimization world, specifically on
1. Computational optimal transport
2. Multiobjective optimization
The lectures on optimal transport will be delivered by Gabriel Peyré, on multiobjective optimization by Gabriele Eichfelder. Each day includes 6 hours of lectures plus coffee breaks.
Attendance
Attendance is free of charge but with a mandatory registration. Lectures are particularly suited for PhD students and young researchers to provide them with the chance of attending two high level courses on continuous optimization, but the school is open to everyone wishing to participate. To register fill this form by 5 May (please, register only when you are sure you are coming: the available classroom is large, so everyone should have a place – in the very unlikely event of too many registrations the priority will not be set upon early booking).
Timetable (preliminary)
Monday 24 June – Gabriel Peyré
Tuesday 25 June – Gabriele Eichfelder
9:00 – 10:30 Lecture I
10:30 – 11:00 Coffee break
11:00 – 12:30 Lecture II
12:30 – 14:00 Lunch break
14:00 – 15:30 Lecture III
15:30 – 16:00 Coffee break
16:00 – 17:00 Lecture IV/Discussion
The courses
Computational optimal transport
by Gabriel Peyré
Optimal transport (OT) is a fundamental mathematical theory that intersects optimization, partial differential equations, and probability. It has recently emerged as a crucial tool for addressing a surprisingly wide range of problems in data science, such as shape registration in medical imaging, structured prediction problems in supervised learning, and training of deep generative networks. This mini-course will intertwine descriptions of the mathematical theory with recent developments in scalable numerical solvers. This approach will underscore the significance of recent advancements in regularized approaches for OT, which make it possible to address high-dimensional learning problems. In the first part of the course, I will explain Monge’s original transportation problem and detail Brenier’s theorem, which guarantees the existence and uniqueness of the optimal map. This theorem is then applied in the contexts of Gaussian distributions and one-dimensional densities. I will then present Kantorovich’s formulation, a convex relaxation that has a strong connection with linear programming. In particular, I will explain the celebrated Birkhoff-von Neumann theorem, which establishes an equivalence between Monge’s and Kantorovich’s formulations. I will also discuss the metric properties of Optimal Transport, which define a metric capable of quantifying the convergence in law of distributions and making it a powerful loss function in imaging and learning. The last part will focus on high-dimensional problems, requiring the use of entropic regularization. I will draw the connection of this approach with the Schrödinger problem in statistical physics and highlight the associated Sinkhorn’s algorithm, which is highly parallelizable on GPUs and has a significant connection with deep learning and neural networks.
Materials for the course, including a small book, slides, and computational resources, can be found online at
https://optimaltransport.github.io/
Multiobjective optimization
by Gabriele Eichfelder
In real-life applications often multiple objective functions arise which have to be optimized at the same time, like costs, energy consumption, and quality. This is known as a multiobjective optimization problem, which involves optimizing a vector-valued objective map. A naïve approach is a weighted sum of the objectives. However, it is difficult to choose appropriate weights beforehand and the values of the individual objective functions can have different scales. Moreover, for non-convex optimization problems, valuable solutions may be overlooked by simplifying with a weighted sum, even if the weights are varied. Thus, several other approaches have been developed to solve these types of problems. The most prominent are nonlinear scalarizations, i.e., the reformulation as a parameter-dependent single-objective replacement problem like the epsilon-constraint method. But also direct methods for special classes of multiobjective problems using for instance descent directions or branch-and-bound techniques have been proposed in the last decades.
These lectures provide an introduction to multiobjective optimization. They begin by presenting the basic optimality concepts and the necessary theoretical background. The focus is then on numerical methods for solving such problems. The lectures give the classical scalarization approaches and discusses their advantages and limitations in terms of quality guarantees as well as for specific classes of optimization problems. A further topic will be descent methods, for which we examine optimality conditions like stationarity. The course continuous with additional ingredients for direct methods for solving multiobjective optimization problems. Participants will gain the ability to select appropriate solution approaches for specific multiobjective optimization problems and critically evaluate the outcomes of standard methods.
The lecturers
Gabriel Peyré (CNRS et École Normale Supérieure, Paris)
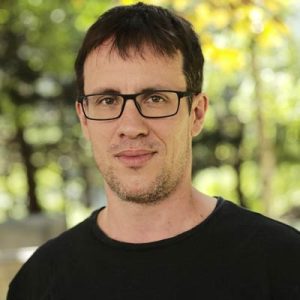
Gabriele Eichfelder (Technische Universität Ilmenau)
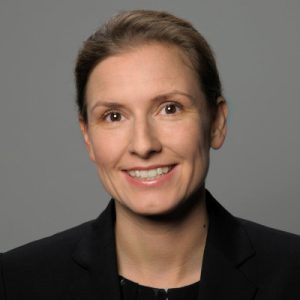
Gabriele Eichfelder is a full professor of Mathematical Methods of Operations Research at the Institute of Mathematics, Technische Universität Ilmenau, Germany. She earned her doctoral degree in 2006 and completed habilitation at the Department of Applied Mathematics at the University of Erlangen-Nuremberg in 2012. She works in the field of Mathematical Optimization with a special interest in nonlinear optimization with vector-valued and set-valued objective functions. In addition to fundamental theoretical studies, she has also been working on numerical solvers for applied engineering problems. Professor Eichfelder has authored two Springer research monographs, has published extensively in top international journals, and has received several publication awards. Recently, she has been elected program director of the SIAM Activity Group on Optimization. Moreover, she served as a member of the program committee for many conferences, including the EURO Conference 2021 in Athens and the recent EUROPT conferences, and as a member of the organizing committee of the 2021 SIAM conference on optimization. She serves on the editorial boards of leading optimization journals, and as an area editor of the Journal of Optimization Theory and Applications. She has been elected EUROPT Fellow in 2024.
Organisation